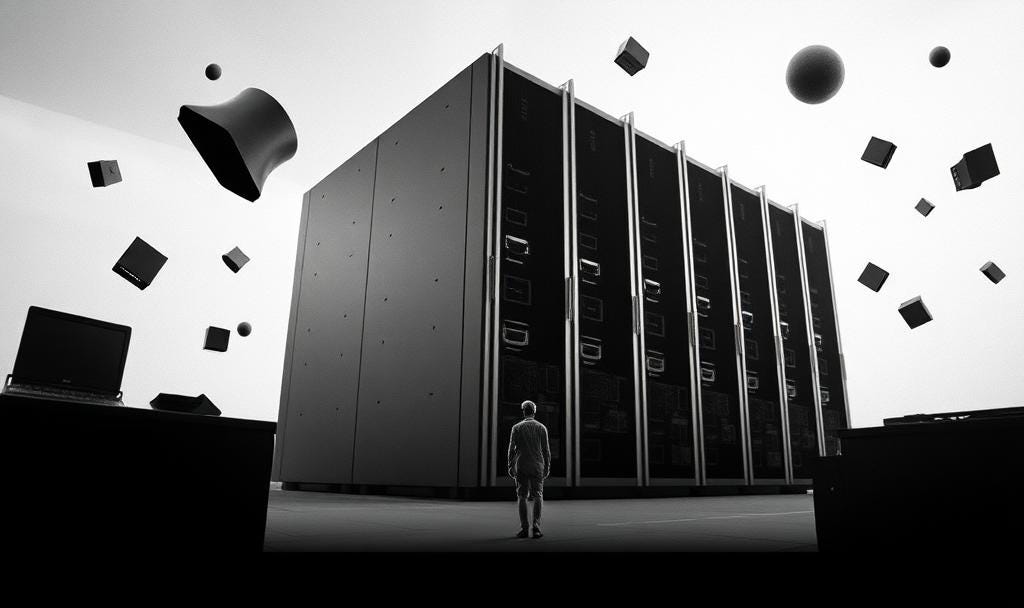
AI is not just another feature—it’s a fundamental shift in how products are built, delivered, and scaled. Yet, most AI product strategies fail before they even start.
Why?
Because companies either treat AI like magic (overhyping its potential) or treat it like a standard software feature (ignoring its unique challenges).
If you want AI to actually drive business impact, you need a real strategy. Here’s how.
1. AI is Not a Feature, It’s a System
Most companies make the mistake of bolting AI onto an existing product, expecting it to "just work." It won’t. AI is a system-level transformation, not a UI tweak.
AI changes how users interact with your product.
AI changes how data flows through your system.
AI changes how decisions are made, both by users and internally.
What to do instead:
Define the role of AI in the user experience (assistive, autonomous, or generative?).
Architect for adaptability, your AI models will evolve, so your product needs to keep up.
Design for uncertainty,
AI is probabilistic, not deterministic, which means failure cases must be part of your strategy.
2. AI Without a Data Strategy is a Science Experiment
AI is only as good as the data you feed it. If you don’t have a plan for collecting, structuring and refining data, your AI product is doomed.
Do you have enough high-quality, labeled data?
Can you continuously improve your datasets as the product scales?
Are you considering privacy, bias, and compliance from the start?
What to do instead:
Start with a data moat. What unique data do you have that others don’t?
Design feedback loops. AI improves over time but only if you set it up to learn from real usage.
Make bias detection a core feature. AI that doesn’t work for everyone is a product liability.
3. AI Monetization: Free Toy or Revenue Driver?
AI is expensive. If your business model is "we’ll figure it out later," you’re already behind.
Here’s where AI actually drives value:
Personalization & efficiency, Making existing workflows 10x better.
Net-new capabilities, Creating new product categories that weren’t possible before.
Ecosystem lock-in, AI that gets better with user data becomes a competitive moat.
What to do instead:
Align AI directly to revenue. If it doesn’t move key business metrics, rethink it.
Don’t offer AI for free unless it leads to paid features.
Plan for AI infrastructure costs. Scaling AI isn’t cheap, make sure your unit economics hold up.
4. Build for Trust: AI is Useless if Users Don’t Trust It
The biggest mistake AI product teams make? Assuming users will trust AI just because it works.
They won’t.
Users need transparency, control and fallback options before they’ll rely on AI-driven decisions.
What to do instead:
Show your work, explainability matters.
Give users control, allow overrides and manual adjustments.
Handle edge cases gracefully, nothing kills trust like an AI that confidently delivers the wrong answer.
5. The AI Playbook: Build Smarter, Scale Faster
Winning AI product teams follow this playbook:
✅ Start with a problem, not a model. Solve real pain points before worrying about tech.
✅ Prototype fast, but launch carefully. AI failures can be costly—iterate aggressively but scale cautiously.
✅ Invest in post-launch monitoring. AI isn’t "done" at launch—it needs constant tuning.
✅ Align AI to business goals. If AI isn’t driving measurable impact, kill it.
Final Thought: AI is a Means, Not the Goal
Your customers don’t care about AI. They care about faster, smarter, better experiences. The best AI product strategies keep that at the center of everything.
🚀 Stop overthinking. Start executing.